Unveiling the Power of Annotation Tools for Machine Learning
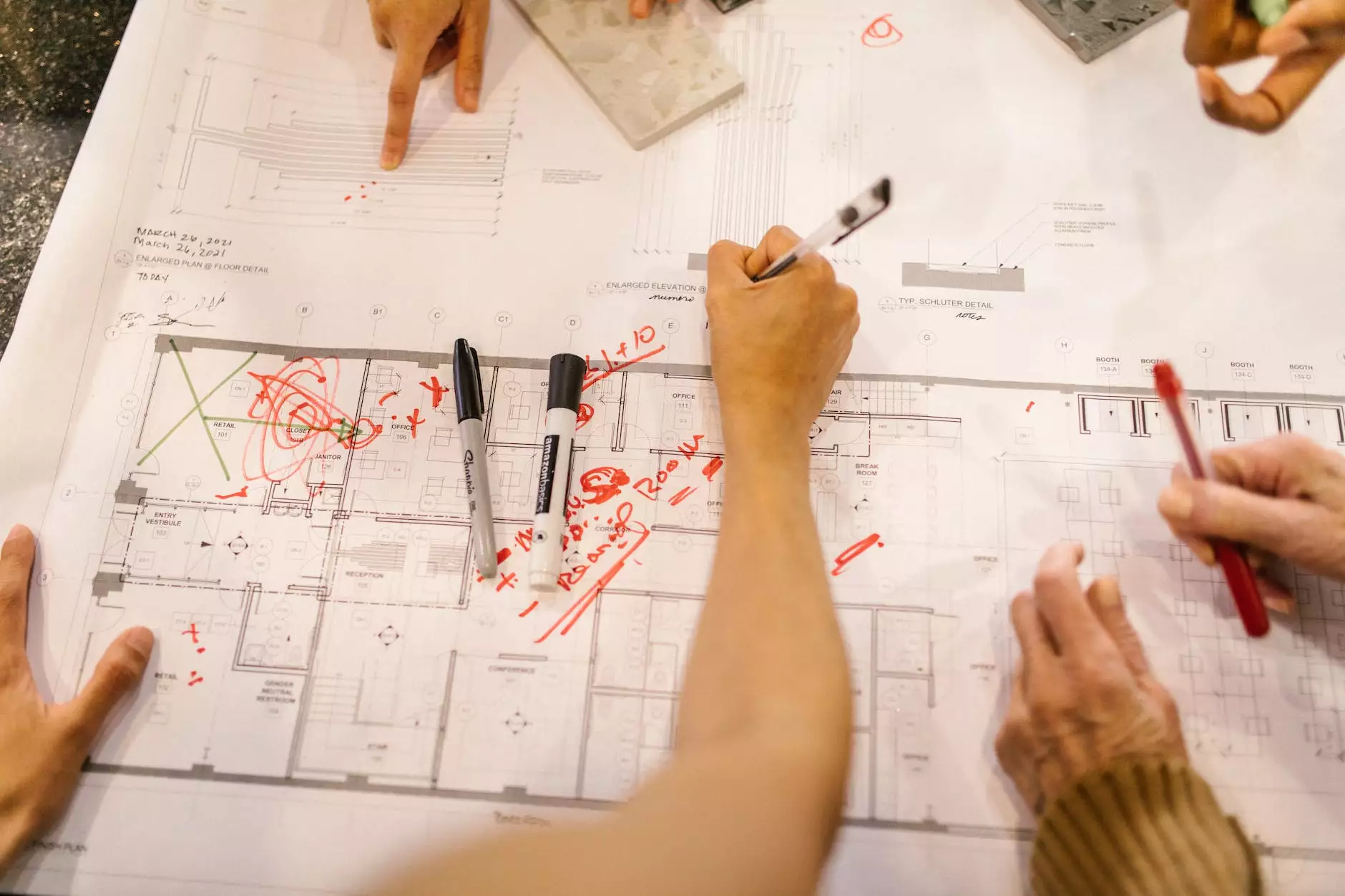
In the rapidly evolving realm of technology, machine learning has emerged as a transformative force, driving innovations across various sectors. The backbone of successful machine learning applications lies in the quality of data fed into them. One crucial aspect of preparing this data is the annotation process, which can significantly enhance the performance of machine learning models. In this article, we will delve into the importance of annotation tools for machine learning, discussing their types, applications, and how they can benefit businesses, especially those within the Home Services and Keys & Locksmiths sectors offered by keymakr.com.
Understanding Annotation in Machine Learning
Annotation refers to the process of labeling data to make it understandable for machine learning algorithms. This step is pivotal because algorithms learn from annotated data, and the accuracy of their predictions heavily relies on the quality and consistency of these annotations. There are several types of data that can be annotated, including:
- Text: Annotating documents, tweets, or customer reviews for sentiment analysis.
- Images: Labeling objects or features within images for image recognition tasks.
- Audio: Transcribing spoken words or annotating audio clips for speech recognition.
- Video: Identifying actions or events in video footage for analysis and prediction.
The effectiveness of machine learning models vastly improves when they are trained on well-annotated datasets. Thus, investing in high-quality annotation tools is essential for businesses aiming to leverage machine learning technologies.
The Significance of Quality Annotation Tools
Quality annotation tools can significantly streamline the process of preparing datasets. Here are a few reasons why businesses should prioritize annotation tools for machine learning:
- Improved Data Accuracy: High-quality tools reduce the chances of errors during the annotation process, leading to more reliable datasets.
- Efficiency and Speed: Advanced annotation tools often come with automation features that accelerate the labeling process.
- Scalability: Many annotation tools can handle large volumes of data, making them perfect for scalable machine learning projects.
- Collaboration Features: These tools often support multi-user collaboration, allowing teams to work together seamlessly.
Types of Annotation Tools for Machine Learning
Various annotation tools for machine learning are available, each catering to specific types of data. Below are several categories of annotation tools commonly used in the industry:
1. Image Annotation Tools
Image annotation tools allow users to label various elements within images. Common tasks include:
- Bounding Box: Drawing rectangles around objects in images.
- Semantic Segmentation: Classifying each pixel in an image into predetermined categories.
- Polygon Annotation: Accurately outlining complex shapes within images.
Tools like Labelbox and VGG Image Annotator are popular choices for image annotation.
2. Text Annotation Tools
Text annotation tools are crucial for labeling text data, which can include annotating entities, parts of speech, or sentiment. Some well-regarded tools are:
- Prodigy: An annotation tool that uses active learning techniques to assist in labeling.
- DocAnnotate: An online tool for creating annotations on documents efficiently.
3. Audio Annotation Tools
Audio annotation tools help transcribe, label, or segment audio data for speech recognition and other applications. Notable tools include:
- Audacity: A widely used audio editing tool that also allows for basic audio annotation.
- IBM Watson Speech to Text: A powerful tool that incorporates machine learning for efficient audio label processing.
4. Video Annotation Tools
Video annotation tools aid in identifying and labeling specific actions within video clips. Some leading video annotation tools are:
- VIA (VGG Image Annotator): A versatile tool allowing users to annotate video frames.
- Scale AI: A platform that streamlines video annotation for various machine learning projects.
Choosing the Right Tool for Your Business Needs
When selecting the most suitable annotation tools for machine learning for your business, consider the following factors:
- Data Type: Choose tools that specialize in the specific type of data your project requires (text, images, audio, or video).
- Team Collaboration: Ensure the tool facilitates seamless collaboration among team members, especially for larger projects.
- Automation Capabilities: Look for tools that offer automation features to enhance efficiency.
- Integration with Other Tools: Your chosen tools should integrate well with your existing workflow and platforms.
Application of Annotation Tools in Home Services and Locksmiths
Businesses in the Home Services and Keys & Locksmiths categories, such as keymakr.com, can greatly benefit from the effective use of annotation tools for machine learning. Here’s how:
Enhancing Customer Service
Through text and voice annotation tools, companies can analyze customer interactions. By labeling sentiment in reviews or support calls, businesses can improve their service and respond better to customer needs.
Marketing Insights
Using image and video annotation can help companies understand customer preference trends through analysis of visual content. Annotating images of products or services can provide insights into what appeals most to consumers.
Improving Security Features
In the locksmith domain, video annotation tools can aid in the analysis of security camera footage. Analyzing events or actions can help in creating more effective security systems and providing better service to customers.
Implementing Annotation Tools: Best Practices
To fully leverage annotation tools for machine learning, consider implementing the following best practices:
- Train Your Team: Ensure your team is well-versed in using the annotation tools efficiently.
- Set Clear Guidelines: Establish clear guidelines for annotation to maintain consistency across datasets.
- Review and Validate: Regularly review annotated data to catch errors and improve quality.
- Iterate on Feedback: Use feedback from machine learning models to refine annotation processes continuously.
Conclusion: The Future of Annotation Tools in Machine Learning
As machine learning continues to expand into every sector, the need for effective and efficient annotation tools for machine learning will only grow. Companies like keymakr.com that invest in high-quality annotation processes will not only improve their machine learning outcomes but also enhance overall business efficiency and customer satisfaction.
The future of business intelligence lies in robust annotated datasets, and organizations that embrace this trend will be at the forefront of innovation. By understanding and implementing sophisticated annotation strategies, businesses can anticipate and respond effectively to market demands, ultimately achieving greater success in their respective fields.